
RFDbaseâ„¢
THE INDUSTRY’S LARGEST ROCK, FLUID, AND PVT DATABASEÂ
SOURCE ROCK – OIL – GAS – PVT – WATER – XRD – XRF – ICP-MS - TRUSAT
If you are a current RFDbase subscriber, or you would like to register for demo access, please click the login button to enter the RFDbase homepage.
GeoMark Research’s on-demand rock, fluid, and PVT data delivers data-driven decisions for discovering and managing earth’s natural resources.
For the past 30 years, this exclusive, curated dataset, has been trusted by energy professionals around the world. Just as our industry evolves to meet new energy demands, RFDbase continues to grow in depth and breadth. From hydrocarbon production to critical mineral exploration, trust RFDbase to provide the quality data you need

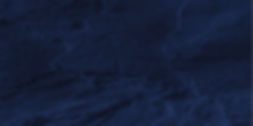
The GeoMark Research Advantage
Integrate your proprietary data with ours to create quality data science trends for rock and fluid properties, spatial mineralogy, and operational vs geological analysis.
Eliminate the hassle of finding, gathering, and cleansing data. Gain immediate access to a trustworthy dataset comprised of more than 500,000 oil/gas/rock samples taken from every major petroleum basin in the world.
Discover basin outliers and other characteristics that can be harnessed as opportunities for growth.Â
Be a hero! Help your explorationists, geochemists, and drilling, reservoir, and facilities engineers work as a team with the right data in a centralized location. Get everyone running from the same playbook!
Access quick-look analog assessments and play-based analysis developed by GeoMark Research’s technical experts to optimize your team’s time and resources.
Gain on-demand access to hydrocarbon properties of more than 16,000 oils from every major basin in the world for immediate economic analyses and facilities planning.
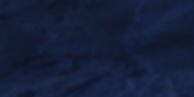
Data in action
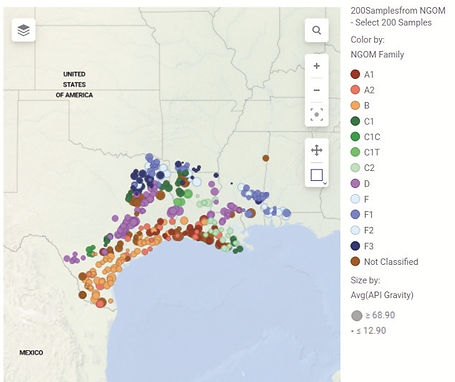
Oil Family Map
This type of map represents the selected oils’ family definition, which is described more fully in a detailed, written report. In summary, these families are defined by Principal Component Analysis (PCA) and Hierarchical Cluster Analysis (HCA). The purpose of these unsupervised machine learning techniques is to best explain the variability of the geochemical data and understand which samples are most similar (or different) from each other and why.